Rare cell populations play a pivotal role in the initiation and progression of immune processes and complex diseases. Our research aims at identification and molecular characterization of such subpopulations in the complex tissue context across health and disease states. Ultimately, we aim at elucidating intra- and intercellular mechanisms of such cell subsets conferring their function and association to organism-level phenotypes. Such insights will enable and drive a new kind of trials that are based on single-cell profiling and achieve cell identity biomarkers, i.e. biomarkers defined by cell subpopulations with characteristic molecular or morphological profiles. To this end, we follow an integrated experimental and machine learning approach that centers around information rich, high dimensional single cell measurements and their comprehensive mathematical analysis in the context of immune and cancer biology.
Rare cell populations
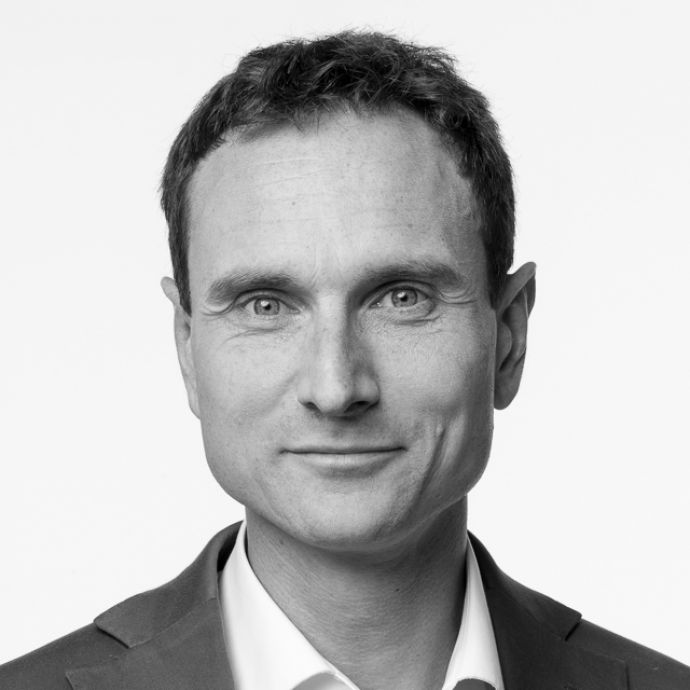
Head of the research group
Publikationen: Publikationen
Biosketch
Prof. Dr. Manfred Claassen has been appointed as Professor for Clinical Bioinformatics at the Medical Faculty of the University of Tübingen since January 2020. Before joining the University of Tübingen he was an Assistant Professor for Computational Biology at the ETH Zürich.
He has carried out parallel studies in Biochemistry and Computer Science at the University of Tübingen and been awarded a Diploma in Biochemistry in 2004 and a Diploma in Computer Science in 2006. In 2010 he obtained a PhD from ETH Zurich. During his doctoral studies he developed statistical methods to design and validate proteome measurements. In 2011 he moved on for postdoctoral training with Daphne Koller at Stanford University, where he focused on inferring informative network models from single cell resolved perturbation studies.
Manfred Claassen’s research focuses on learning explainable quantitative models in systems medicine from high dimensional single-cell omics data. Health and disease status of multicellular organisms pivotally depends on rare cell populations, such as hematopoietic stem cells or tumor initiating cell subsets. The Claassen lab develops representation learning approaches to identify disease associated cell subsets, ultimately enabling comparative single-cell studies, e.g. for immunotherapy response prediction in melanoma or diagnosis of multiple sclerosis and detection spatial tissue determinants of disease association. Particular focus lies on dynamic processes which are increasingly studied by high-dimensional single-cell snapshots. Such measurements enable evaluation of cell population distributions and their evolution over time.
Selected publications
- Ruf B, Bruhns M, Babaei S, … , Claassen M*, Korangy F*, Greten TF*, Tumor-Associated Macrophages Trigger MAIT Cell Dysfunction at the HCC Invasive Margin, Cell, accepted, 2023.
- Gupta R, Cerletti D, Gut G, Oxenius A, Claassen M*, Simulation based inference of differentiation trajectories from RNA velocity fields. Cell Reports Methods, 2(12):100359, 2022.
- Otesteanu CF, Ugrinic M, Holzner G, Chang YT, Fassnacht C, Guenova E, Stavrakis S, deMello A, Claassen M*, A weakly supervised deep learning approach for label-free imaging flow-cytometry-based blood diagnostics, Cell Reports Methods, 1(6), 100094, 2021
- Kopf A, Fortuin V, Somnath VR, Claassen M*, Mixture-of-Experts Variational Autoencoder for clustering and generating from similarity-based representations on single cell data, PLoS Computational Biology, 17 (6), e1009086, 2021
- Sandu I, Cerletti D, Borsa M, Spadafora I, Welten SPM, Stolz U, Oxenius A*, and Claassen M*, Landscape of exhausted virus-specific CD8 T cells in chronic LCMV infection. Cell Reports. 2020.
- Taylor-King JP, Riseth A. Macnair W., Claassen M*. Dynamic Distribution Decomposition for Single-Cell Snapshot Time Series Identifies Subpopulations and Trajectories during iPSC Reprogramming. PLOS Comput Biol. 2020;16(1):e1007491.
- Galli E, HartmannFJ, Schreiner B, Ingelfinger F, Arvaniti E, Diebold M, Mrdjen D, van der Meer F, Krieg C, Nimer FA, Sanderson N, Stadelman C, Khademi M, Piehl F, Claassen M, Derfuss T, Olsson T, Becher B. GM-CSF and CXCR4 define a T helper cell signature in multiple sclerosis. Nat Med. 2019;25(8):1290-1300.
- Macnair W, De Vargas Roditi L, Ganscha S, Claassen M*. Tree-ensemble analysis assesses presence of multifurcations in single cell data. Mol Syst Biol. 2019;15(3):e8552.
- Arvaniti E*, Fricker K*, Moret M, Rupp N, Hermanns T, Fankhauser C, Wey N, Wild PJ, Rüschoff J*, Claassen M*. Automated Gleason grading of prostate cancer tissue microarrays via deep learning. Sci Rep. 2018;8(1):12054.
- Arvaniti E, Claassen M*. Sensitive detection of rare disease-associated cell subsets via representation learning. Nat Commun. 2017;8:14825.